Hiring Top Artificial Intelligence Talent: Must-Know AI Engineer Interview Questions and Answers
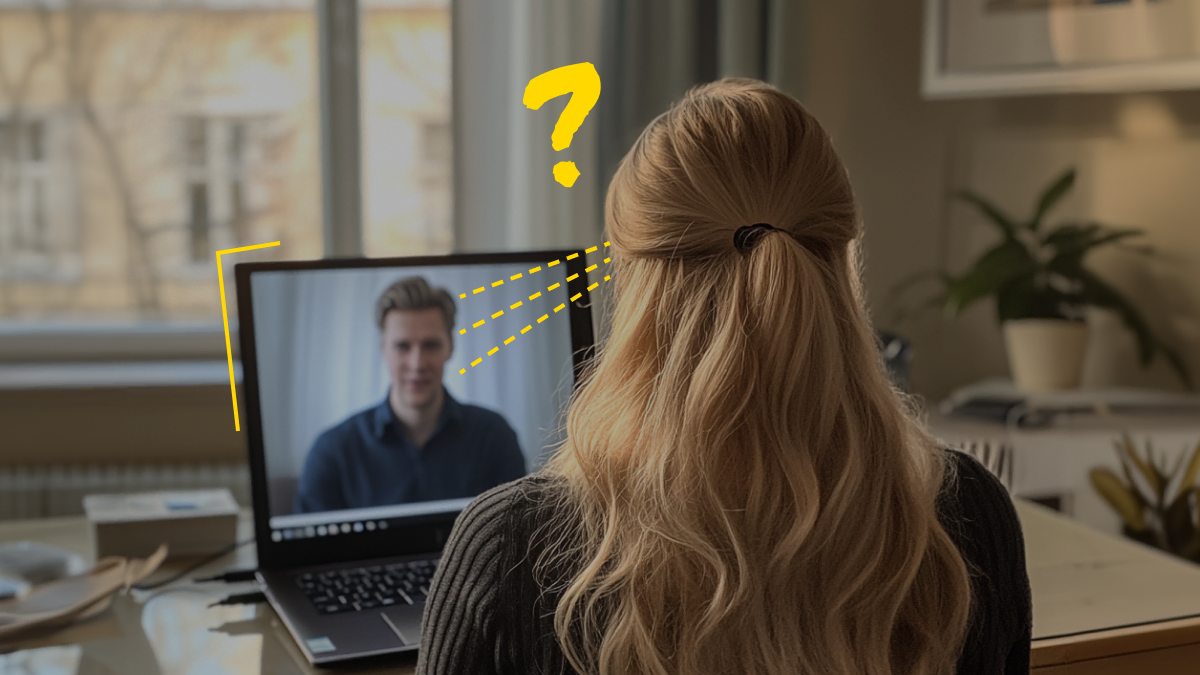
- Before AI Engineer Screening: Selecting the Right Format
- Part 1. Background Check Questions
- Part 2. General Interview Questions
- Part 3. Technical Interview Questions
- Part 4. Scenario-Based Questions
- Part 5. Behavioral and Team-Based Questions
- Part 6. Extra Questions for Senior Engineers
- Interview Assessment: Candidate Evaluation Cheatsheet
- Best Practices to Use When Interviewing Artificial Intelligence Experts
- ML Engineer Hiring: Do It In-House or Find an IT team Extension Vendor ?
Soaring global demand for skilled AI engineers brings a new focus for HR. The current landscape demonstrates several rapid shifts calling for intensive recruitment of artificial intelligence experts:
- The demand for Gen AI roles has increased by 500%, according to a report by The Sun, and AI expert jobs are expected to remain among the top 10 fastest-growing positions between 2024 and 2027.
- Businesses that have already adopted AI are experiencing almost a five times labor productivity boost as stated in the PwC’s 2024 Global AI Jobs Barometer, meaning the demand for AI experts will keep growing.
AI adoption surged to 72% in 2024, with sectors like healthcare, manufacturing, and IT leading the way (McKinsey & Company).
Before AI Engineer Screening: Selecting the Right Format
Without a well-thought-out selection process, finding the right candidate can be challenging. The choice depends on various factors, from project requirements and business priorities to communication principles within your organization.
Check out the table below to identify the right-fit approach for your specific project.
Format | Description |
Candidate interview (30-45 minutes) | Purpose: Assessing the candidate’s AI knowledge, experience, and role interest. |
Whiteboard / live coding session (1-2 hours) | Purpose: Evaluating the candidate’s problem-solving, coding skills, and performance under pressure. |
Home assignment (1-7 days deadline) | Purpose: Understanding the candidate’s approach to real-world projects and skill level. |
Portfolio review and project presentation (1-1,5 hours) | Purpose: Evaluating the candidate’s proficiency based on past projects, with a focus on their ability to explain AI concepts to both technical and non-technical stakeholders. |
For the most effective recruitment process, consider combining several screening steps to evaluate the candidate’s skills from different angles and make a well-rounded decision about the right-fit candidate.
In addition, you can adjust the process as per your preferences — either by combining or splitting the screening process into multiple sessions. The choice depends on your company’s internal workflow preferences, the complexity of the role, and the depth of evaluation.
Artificial Intelligence Interview Questions: A Complete List
Moving on to the AI interview questions and answers: while candidate responses might be unpredictable, there are ways to get ready for efficient response analysis during the meeting.
The below-mentioned detailed breakdown of interview questions for AI engineer illustrates the complexity of the entire process — while focusing on some crucial assessment aspects that might otherwise be missed. So, buckle up to explore the behind-the-scenes of the Mobilunity strategy and core recommendations compiled by our experts.
Part 1. Background Check Questions
This job interview cluster aims at understanding the candidates’ educational background, previous work experience, and specific projects they have undertaken.
🤖 “Can you walk us through your career journey and what led you to the field of AI?”
🟢Green flags to look for in a candidate:
- Clear, linear career timeline.
Demonstrating a clear path of progression from early roles to key milestones.
- The use of STAR framework when answering.
Following the response structure as Situation, Task, Action, and Result.
Example of a good answer:
“I started my career as a software developer and was later introduced to AI and machine learning through a predictive analytics project. My role there was combining collaborative filtering with content-based methods to ensure higher model accuracy. This strategy proved successful since we were able to demonstrate significant improvements for the client. As I moved on with my career, I got more involved in conversational agents and speech recognition projects. For example, my latest work was creating a virtual assistant for a fintech startup.”
What to avoid:
❌ Generic reasons without personal connection.
❌ Emphasis on salary or prestige without deeper passion.
Optional Questions
- What is your educational background? Do you have any certification in artificial intelligence in particular?
- What industries have you worked in, and how has your experience in those industries shaped your approach to artificial intelligence and machine learning?
Part 2. General Interview Questions
This list of general AI interview questions is aimed to ensure that candidates not only have the technical skills necessary for the role but also possess the right mindset and values to thrive within the company.
2.1. On Motivation and Career Goals
🤖 “What motivated you to pursue a career in the AI-related field?”
🟢Green flags to look for in a candidate:
- Clear passion.
Demonstrating genuine interest in the field with specific motivating experiences.
- Relevance.
Connecting motivation to the job role responsibilities. - Practical examples.
Providing relevant project or mentor experiences.
Example of a good answer:
“Emerging technologies have always piqued my interest, especially in how they can address complex problems in new ways. The moment I started working towards developing solutions based on artificial intelligence, I was struck by the potential of artificial intelligence.
There are so many things that can be done with it: for example, through machine learning we can forecast health outcomes — or save easily hundreds of hours that would have been consumed by routine operations. This makes your work not only mind-challenging and exciting, but also highly rewarding and impactful.”
What to avoid:
❌ Generic reasons without personal connection.
❌ Emphasis on salary or prestige without deeper passion.
❌ No relevant expertise or qualifications.
🤖 “What are your long-term career goals in this field, and how would you like to continue growing?”
🟢Green flags to look for in a candidate:
- Emphasis on adaptability and innovation.
Showing a willingness to master the ever-evolving and emerging artificial neural network technologies.
- Realistic goals.
Setting career goals that align with the current skill set and experience.
- Сontinous learning attitude.
Demonstrating eagerness to explore new tech advancements.
Example of a good answer:
“The area of natural language processing and AI-driven customer support tools is particularly interesting to me. I plan to gain expertise in dialogue systems and voice recognition. Also, I am yet to enroll in the advanced course in NLP and machine learning. As a long-term vision, I aim at leading large-scale project teams or even to create my own AI-powered startup company.”
What to avoid:
❌ Lack of clarity or professional direction.
❌ Lack of growth mindset.
❌ Overemphasis on expected individual contributions.
Optional Questions
- How have your career goals evolved since you first started working in the field?
- Is there a particular AI-related problem or challenge that you are personally passionate or curious about solving?
2.2. On Cultural Fit
🤖 “How do you approach working with other team members and stakeholders?”
🟢Green flags to look for in a candidate:
- The value of engagement.
Recognizing the need to consider both technical and non-technical stakeholders.
- Clarity in communication.
Ensuring complex technical information is communicated in a clear, concise manner.
Example of a good answer:
“I make sure everyone understands the project’s goals by breaking down complex technical details into simple terms for non-technical stakeholders, while still keeping things precise for the technical team. As a seasoned team player, I’m flexible with different working styles and always open to feedback, also making sure any important concerns are addressed.”
What to avoid:
❌ Lack of flexibility in communication or project-related processes.
❌ Relying on solo contributions rather than collaboration.
❌ Inability to adapt communication style for various stakeholders.
🤖 “What top 3 values are most important to you in a workplace, and how do they influence your work?”
🟢Green flags to look for in a candidate:
- Alignment with company values and job role specifics.
Emphasizing values that align with both personal and company culture.
- Impact on work.
Demonstrating how these values influence motivation, productivity, or other workplace dynamics.
Example of a good answer:
“First, I highly value curiosity, because it helps me stay ahead of the curve. Accountability is another one — in my view, it’s the key to accurate, reliable, and ethical solutions. Lastly, diligence helps me focus on the details, which is crucial when fine-tuning the models and working with data.”
What to avoid:
❌ Vague, generic answers that do not match real-life scenarios.
❌ No context behind values, inability to clearly explain why they matter.
Optional Questions
- Would you consider yourself flexible when it comes to some specific workspace processes or regulations?
- In what type of company would you feel uncomfortable working, and why?
2.3. On Awareness of Industry Trends
🤖 “How do you stay updated with the latest artificial intelligence trends?”
🟢Green flags to look for in a candidate:
- Mentioning a list of specific resources.
Providing references to AI-oriented media, communities, thought leaders etc.
- Examples of the latest trends.
Highlighting one or several hot news in machine learning and artificial intelligence.
Example of a good answer:
“I follow several tech influencers on YouTube and LinkedIn who review the latest development tools, AI-powered features, and engineering methodologies. I also keep an eye on industry leaders. For example, Jensen Huang, Nvidia’s CEO, frequently shares thought-provoking insights. I also try to weekly monitor the latest publications on real-life projects, especially in areas of natural language processing.”
What to avoid:
❌ Reliance on questionable, unverified data sources.
❌ No clear strategy or approach for staying informed on trends.
Optional Questions
- How do you decide which trends are worth exploring further?
- Is there any recent trend or approach that you find particularly interesting or impactful for machine learning?
Part 3. Technical Interview Questions
This section focuses on the candidate’s tech knowledge and expertise, including the understanding of fundamental AI concepts, implementation pitfalls, and adoption strategies.
3.1. On AI Fundamentals
🤖 “Explain the difference between machine learning, deep learning, and artificial intelligence”
🟢Green flags to look for in a candidate:
- Clarity in definitions.
Explaining relationships between concepts in a structured and concise manner.
- Examples of real-world applications.
Supporting the statements with examples of how each concept is applied in the real world.
Example of a good answer:
“Artificial Intelligence is the broadest concept of the three, encompassing any case where AI performs tasks typically done by humans. AI-powered automation, virtual assistants, generative AI — they can all fall under this category.
Machine Learning is a subset of AI, where algorithms learn from data to make predictions or decisions without being manually programmed for each task. Netflix or Amazon is a good example here.
Meantime, Deep Learning is a subset of machine learning that uses multi-layered neural networks to analyze large datasets and complex patterns, like image or speech recognition used in GPT-4.”
What to avoid:
❌ Overly technical or lengthy explanations that divert from the key points.
🤖 “Supervised, unsupervised, and reinforcement learning: in which cases is each one best?”
🟢Green flags to look for in a candidate:
- Reflections based on personal experience.
Demonstrating firsthand understanding of the concepts.
- Specific examples.
Supporting each statement with real-world application.
Example of a good answer:
“Supervised learning is used when the task is well-defined and labeled data is available, like in the case of identifying financial frauds. Unsupervised learning, in turn, can be helpful for finding underlying structures in data without predefined labels, such as for cybersecurity anomaly detection. Finally, speaking of reinforcement learning, it is useful for trial and error cases — like training a self-driving car to navigate a city.”
What to avoid:
❌ Lack of structure in the answer.
❌ Failure to provide distinct differentiators.
Optional Questions
- Can you explain in simple words what neural networks are and how they work?
- How do you differentiate between feature extraction and feature selection in machine learning?
3.2. On Algorithmic Knowledge
🤖 “How do you decide which machine learning algorithm to use for a specific problem?”
🟢Green flags to look for in a candidate:
- Structured response with a list of criteria.
Clearly articulating the decision-making process and selection factors.
- Insights from personal experience.
Providing specific examples from previous projects.
Example of a good answer:
“I consider several things when selecting a specific algorithm: the type and complexity of the problem, the size and type of data, and what are the task’s top priorities — be it accuracy, interpretability, computational resources, or anything else. For example, if it’s a classification problem, I would use decision trees, logistic regression, or support vector machines. For larger, complex datasets, deep learning models like neural networks work best.
Additionally, I consider the balance between accuracy and interpretability, using decision trees for clear results and CNNs for tasks like image recognition. In many cases, speed matters significantly too, so when it comes to real-time tasks, I choose faster algorithms like k-nearest neighbors.”
What to avoid:
❌ Limited response, explaining only one case scenario.
🤖 “What is overfitting, and how can you prevent it?”
🟢Green flags to look for in a candidate:
- Concise yet clear definition.
Providing a straightforward explanation without unnecessary complexity.
- Real-life application of various prevention techniques.
Highlighting which approaches are ideal for particular cases.
Example of a good answer:
“Overfitting occurs when a model captures both patterns and noise in the training data, leading to poor generalization. I’ve looked for several effective ways to tackle this. For example, I often use regularization (L1/L2) to control complexity, cross-validation to ensure generalization, and early stopping to avoid overtraining. In neural networks, I’ve found dropout effective. For example, in an NLP project, combining dropout with L2 regularization significantly improved the model’s performance on test data.”
What to avoid:
❌ Focus on one technique only.
❌ Lack of practical examples.
Optional Questions
- What are the key differences between classification and regression tasks in machine learning?
- How do you handle missing data in a dataset when training a machine learning model?
3.3. On Practical Implementation
🤖 “Describe your experience with Natural Language Processing (NLP). What tools have you used?”
🟢Green flags to look for in a candidate:
- Evaluation of most common tools.
Providing personal assessment of popular NLP tools.
- Unique NLP considerations or challenges.
Highlighting the need to address language ambiguity, context understanding, and other critical aspects.
Example of a good answer:
“I’ve worked on several projects that required different NLP tools, each with its unique strengths and challenges. For instance, I used NLTK for text preprocessing and tokenization, which was essential for preparing datasets for analysis. NLTK is great for its extensive libraries and flexibility, but it can be slower with larger datasets.
In a more recent project, I leveraged spaCy for named entity recognition to efficiently extract critical information from large volumes of text. SpaCy’s speed and built-in capabilities for dependency parsing were significant advantages, especially when processing real-time data.
Additionally, I used Hugging Face Transformers to develop a sentiment analysis model for analyzing customer feedback on social media, which accelerated our development process and improved accuracy.”
What to avoid:
❌ Theoretical, impractical definition of NLP.
❌ Lack of personal perspective
🤖 “What are Generative Adversarial Networks (GANs) and how have you used them?”
🟢Green flags to look for in a candidate:
- Review of the impact of GANs.
Highlighting the effects of GAN for specific projects or scenarios.
- Understanding of core challenges.
Mentioning mode collapse, training instability, quality of training data, the need for significant computational resources, to name a few.
Example of a good answer:
“Generative Adversarial Networks (GANs) consist of two neural networks — a generator and a discriminator. They work against each other to produce realistic data. The generator creates new data samples, while the discriminator evaluates them against real data. This helps improve the generator’s output over time.
In my experience, I’ve used GANs in several projects. For instance, I applied GANs to generate synthetic images for data augmentation in a computer vision project, which helped provide more training examples and thus improve model performance. I also experimented with conditional GANs to create targeted images based on specific attributes, which was particularly useful in generating diverse outputs for creative applications.
One of the unique challenges with GANs is ensuring the stability of training, as they can often suffer from issues like mode collapse, where the generator produces a limited variety of outputs. I’ve found that carefully tuning hyperparameters and using techniques like progressive growing can help mitigate these issues and lead to more stable training and better results.”
What to avoid:
❌ Theoretical, impractical definition of NLP.
❌ Lack of personal perspective.
Optional Questions
- Can you walk me through a recent AI project where you faced significant implementation challenges?
- Which cloud platforms or services have you used for building and deploying AI models, and what was your experience?
Part 4. Scenario-Based Questions
This section aims to evaluate the candidate’s ability to address real-world challenges, implement AI solutions effectively, and think critically when solving complex problems.
4.1. On AI Application In Real-World Problems
🤖 “You need to develop a recommendation system for an e-commerce platform. How would you approach this?”
🟢Green flags to look for in a candidate:
- Step-by-step explanation.
Providing a structured breakdown of the process.
- Consideration of potential pitfalls.
Mentioning key risks and their prevention techniques.
Example of a good answer:
“When developing a recommendation system for an e-commerce platform, I would start by evaluating the problem type. For personalized recommendations, I would consider using collaborative filtering based on user behavior and preferences.
While considered efficient, this method may have its limitations, such as data sparsity in new user scenarios. To address this challenge, I’d implement content-based filtering alongside collaborative filtering to enhance the diversity of recommendations. Also, I would focus on optimizing the algorithm for efficient real-time processing with tools like TensorFlow or PyTorch.”
What to avoid:
❌ Neglecting data analysis.
❌ Ignoring optimization.
❌ Disregarding the latest tools or techniques.
🤖 “Describe how you would implement a predictive maintenance system in a manufacturing plant.”
🟢Green flags to look for in a candidate:
- Step-by-step implementation plan.
Outlining a structured approach to implementing the system, covering data collection, model selection, and deployment phases.
- Recommendations on specific tools.
Mentioning specific technologies that would be used in the implementation (IoT sensors, data analytics platforms, etc.)
Example of a good answer:
“I would start by installing IoT sensors on critical machinery and setting them up to collect real-time data on parameters — temperature, vibration, operational hours, etc. This data would be integrated into a centralized database, with cleaning techniques applied to ensure accuracy.
Then, I would then select a suitable machine learning model. Regression analysis or classification can be the optimal fit here, using libraries like Scikit-learn or TensorFlow.
Next on, there’s training and model validation through cross-validation based on the historical maintenance data. When it’s done, I would deploy it within the existing maintenance management system, ensuring real-time alerts. The only thing that remains then is monitoring and updates whenever any new insights are gained.”
What to avoid:
❌ Failure to identify key steps.
❌ Not considering user feedback or operational data.
Optional Questions
- How would you design deep learning models to improve customer churn prediction for a telecom company?
- How would you approach building an AI system for detecting fraud in a financial institution?
4.2. On Problem-Solving
🤖 “You encounter a new technology that you’re unfamiliar with, but it’s necessary for a project. How would you go about learning and implementing it?”
🟢Green flags to look for in a candidate:
- Growth mindset mentality.
Expressing openness and curiosity toward new approaches and technologies.
- Diligence and focus on thorough testing.
Highlighting the importance of testing and validating new tools.
Example of a good answer:
“To get acquainted with the unknown AI technology, I review official documentation, tutorials, and community resources like forums and GitHub repositories. After I study it well enough, I would start testing it out on small prototypes or experiments to gain a hands-on understanding of how it actually works.
Once I’m comfortable using it, I adopt an incremental implementation strategy to integrate the technology into the project in phases and monitor the results as I go.”
What to avoid:
❌ Disregard for prior research in favor of solely hands-on testing.
🤖 “How would you avoid the most common pitfalls associated with neural networks in AI?”
🟢Green flags to look for in a candidate:
- Highlights on multiple challenges.
Pinpointing different aspects of potential risks that might occur.
- Hands-on experimentation.
Emphasizing on testing out various strategies.
Example of a good answer:
“Neural networks challenges are many. Take overfitting mentioned earlier, lack of interpretability, data quality issues, training instability, ignoring hyperparameter tuning, computational resources limitations, etc.
The ideal method to address them is through a mix of various strategies. For example, I’d use dropout, regularization, and early stopping to ensure a diverse dataset, prioritize interpretability with tools like LIME or SHAP, focus on thorough data preprocessing, and optimize performance through hyperparameter tuning.
What to avoid:
❌ Disregard of potential risks and their impact.
❌ Overreliance on familiar architectures without adapting to project needs.
Optional Questions
- Can you describe a time when you had to innovate to overcome a major challenge in an AI project? What steps did you take, and what was the outcome?
- How do you ensure that the AI models you develop can adapt to changing requirements or evolving data over time?
Part 5. Behavioral and Team-Based Questions
This interview part evaluates the candidate’s ability to collaborate effectively in dedicated team environments, handle conflicts, and communicate complex AI concepts to both technical and non-technical stakeholders.
🤖 “Provide an example of how you have worked with a cross-functional team to deliver an AI-powered solution.”
🟢Green flags to look for in a candidate:
- Collaboration-oriented mindset.
Being open to feedback and communication with other team members.
- Role clarity.
Demonstrating a clear understanding of responsibilities within cross-functional teams.
Example of a good answer:
“Overall, I am experienced in collaborating with various departments and adapting the level of detail and communication style to match their expertise.
For example, in one of the projects, I collaborated closely with customer service representatives to gather insights on popular queries, which informed our model’s training data. We had regular feedback sessions that allowed us to address challenges quickly and adapt our strategies, which eventually led to project success.”
What to avoid:
❌ Ignoring the importance of user perspective from various backgrounds.
🤖 “How do you handle disagreements in a team setting when choosing AI models?”
🟢Green flags to look for in a candidate:
- Structured approach to decision-making.
Describing a systematic process for evaluating options based on data, collective input, and expertise.
- Focus on the common goal.
Emphasizing the importance of keeping the team aligned on the project’s objectives and ensuring that decisions serve the overall project’s success.
Example of a good answer:
“In one of the projects, I worked with a cross-functional team to develop a natural language processing tool for a retail company that wanted to automate their customer support. Our team included NLP specialists, software engineers, and customer service representatives. For this project, I collaborated closely with customer service representatives to gather insights on common queries, which informed our model’s training data. We had regular feedback sessions that allowed us to address challenges quickly and adapt our strategies.”
What to avoid:
❌ Vague or generic responses lacking specificity or real-life scenarios.
❌ Not mentioning potential decision-making criteria.
🤖 “Describe a time when you had to explain a complex AI concept to a non-technical audience. How did you approach it?”
🟢Green flags to look for in a candidate:
- Openness to feedback and discussion.
Emphasizing the importance of team alignment and ensuring that decisions serve the overall project success.
- Structured approach to decision-making.
Describing a systematic process for evaluating options based on data, collective input, and expertise.
Example of a good answer:
“In a recent project developing a machine learning model, our cross-functional team had differing opinions on algorithm selection. We facilitated a brainstorming session where each member presented their rationale. We focused on jointly discussing the key criteria like accuracy, computational efficiency, and scalability. In the end, we were able to reach a consensus that combined our ideas, resulting in a more robust model.”
What to avoid:
❌ Vague or generic responses that lack specificity or real-life scenarios.
❌ Not mentioning potential decision-making criteria.
Optional Questions
- Do you prefer working independently or in close collaboration with a team?
- Do you have any communication principles or preferences that you follow when working as a part of a team?
Part 6. Extra Questions for Senior Engineers
These top AI interview questions for senior specialists are designed to evaluate the candidate’s proficiency level and the ability to lead project teams effectively.
6.1. On Model Optimization and Scalability
🤖 “What methods do you use to optimize the neural network model performance?”
🟢Green flags to look for in a candidate:
- Holistic perspective.
Illustrating the ability to synthesize various techniques to enhance the overall model effectiveness.
- Adaptability and continuous improvement mindset.
Showcasing a willingness to experiment with various techniques with a strong focus on ongoing evaluation and iteration.
Example of a good answer:
“As a senior developer, I optimize the model performance through several key methods: I systematically adjust hyperparameters using grid or random search with tools like Optuna or Hyperopt. Plus, I focus on feature engineering to enhance accuracy through normalization, one-hot encoding, and interaction features.
To prevent overfitting, I apply regularization techniques such as L1 and L2. I also implement ensemble methods like bagging and boosting, and explore advanced architectures like transformers for NLP or CNNs for image processing. After deployment, I continuously monitor performance metrics like precision, recall, and F1 score, just in case there are any issues to address.”
What to avoid:
❌ Limiting the approach to just one solution rather than leveraging a comprehensive approach.
❌ Disregarding iterative improvements post-deployment.
🤖 “Describe how you handle model drift in a production environment.”
🟢Green flags to look for in a candidate:
- Clear Understanding of drift indicators.
Identifying specific signs of model drift and explaining how they prompt further investigation or action.
- Focus on impact.
Demonstrating how specific approaches affect business outcomes.
Example of a good answer:
“To handle model drift, I monitor various performance metrics: accuracy, precision, recall over time, and others. In doing so, I regularly assess input data for changes in distribution compared to the training data. I also establish performance baselines to quickly identify declines.
When drift is confirmed, I gather new training data that reflects the current environment and retrain the model. For such cases, transfer learning can be applied for quicker adaptation.”
What to avoid:
❌ Disregarding the essential need for regular monitoring.
❌ Reactive approach, waiting for performance issues to arise before taking action.
Optional Questions
- What techniques do you use to minimize latency in real-time deep-learning applications?
- What strategies would you suggest applying to optimize memory usage in large models?
6.2. On Ethics and Bias
🤖 “How do you ensure your AI models are fair and unbiased?”
🟢Green flags to look for in a candidate:
- Solution-oriented, comprehensive approach.
Addressing multiple facets of ethical considerations, from transparency to the societal implications and beyond.
- Commitment to fairness.
Reflecting a deep understanding and commitment to mitigating biases.
Example of a good answer:
“Each of these considerations can be approached in various ways. For example, to reduce bias, it’s essential to implement diverse and representative training datasets. To clarify decision-making processes and enhance transparency, I’d use explainability tools like LIME or SHAP, and so on.”
What to avoid:
❌ Vague or generic responses that lack specificity.
❌ Ignoring the importance of user perspective from various backgrounds during AI development process.
Optional Questions
- In your view, what strategies can be applied to ensure people don’t use AI in unintended ways?
- How can AI/ML project teams evaluate the long-term social and economic impacts of the solutions they develop?
6.3. On Leadership and Strategy
🤖 “How do you mentor your junior team members?”
🟢Green flags to look for in a candidate:
- Commitment to mentorship and its results.
Articulating approaches that prioritize the mentee’s development and align with their career goals.
- Open communication.
Describing the ways to foster a culture where questions are welcomed.
Example of a good answer:
“My key goal is to create a supportive environment that encourages their growth. I believe in the value of hands-on experience, so I guide them through real-world projects. I make it a point to provide constructive feedback on their work, emphasizing their strengths and areas for improvement.
I believe there should always be room for open communication and questions. Besides, I also have a collection of resources to share that they may find valuable in advancing their skills.”
What to avoid:
❌ Limited engagement and lack of regular feedback.
❌ Relying solely on trial and error strategy when mentoring junior engineers.
Optional Questions
- How’s your experience of having to lead a team through a major project pivot? How did you manage the transition?
- How do you approach risk management in deep learning projects, particularly in ensuring model reliability?
Interview Assessment: Candidate Evaluation Cheatsheet
To help you streamline the evaluation process and assess the candidate’s skills from multiple angles, we recommend using the below-mentioned form, tailor-made by Mobilunity experts for AI-specialized engineering roles.
Best Practices to Use When Interviewing Artificial Intelligence Experts
Whether you are a novice or a skilled interviewer, keeping these principles in mind will help you guide recruitment efforts:
> Understand the key role requirements and the unique nature of a specific project, be it machine learning, NLP, deep learning, computer vision niche, or specialized tools like SAP AI developers certification, or AI Project Management.
> Ensure a multi-faceted assessment by balancing technical questions with soft skill evaluations, such as teamwork, adaptability, and problem-solving.
> Assess the candidate’s practical application of the AI skills by simulating relevant real-life scenarios.
> Consider the cultural fit and candidates’ ability to work within your company’s culture.
Pro Tip:
Look for those who can not only discuss technical details but also сlearly articulate the business impact of their solutions — it means they have a profound understanding of their role.
ML Engineer Hiring: Do It In-House or Find an IT team Extension Vendor ?
Due to the highly specialized nature of artificial neural network expertise, recruiting such specialists requires a more thorough evaluation of technical depth, hands-on experience, problem-solving ability, and interdisciplinary collaboration than other roles.
This makes dedicated AI-focused engineers a more cost-effective option. Additionally, there are other factors to consider when making your decision. Let’s explore them.
1. Talent gap driven by high demand.
Unlike companies that may struggle to find the right candidate, IT staff augmentation agencies access a wider talent pool across regions and are able to address talent shortages more effectively.
2. High recruitment costs.
Working with a dedicated dev team vendor can help reduce the total costs of hiring in-house engineers, which can be particularly high in the U.S. and Western Europe. Recruitment, onboarding, and benefits can add up to $150,000 to $250,000 per hire if hire in house.
3. Lengthy recruitment process.
Finding the right talent can take months — the role’s complexity and multiple selection rounds add an extra challenge to it. In contrast, hiring an IT team extension vendor can speed up the process with pre-vetted candidates ready to deploy.
4. Scalability.
As projects grow, scaling your in-house team quickly can be challenging. Dedicated development team agencies, in turn, can help you scale up or down without further complications or long-term commitments.
5. Employee churn rates.
The surge in demand for AI-proficient engineers has made the market highly competitive, increasing the risk of losing your current team members to other companies.
Team augmentation vendors dedicate extra effort to reduce such churn risks through HR strategies, compensation, and social packages. Moreover, even if your employee leaves, they are able to provide replacements within a short period of time.
Finding the right AI engineer is essential for labor productivity enhancements and overall process efficiency. A thorough interview process will help you identify top talent and create a strong foundation for future achievements. At Mobilunity, we specialize in connecting businesses with skilled AI professionals who align with your unique needs. Whether you’re expanding your AI capabilities or starting new projects, our team is here to help you build the expertise you need.
Disclaimer: All salaries and prices mentioned within the article are approximate numbers based on the research done by our in-house Marketing Research Team. Please use these numbers as a reference for comparison only. Feel free to use the contact form to inquire on the specific cost of the talent according to your vacancy requirements and chosen model of engagement.