Conversational AI in Banking
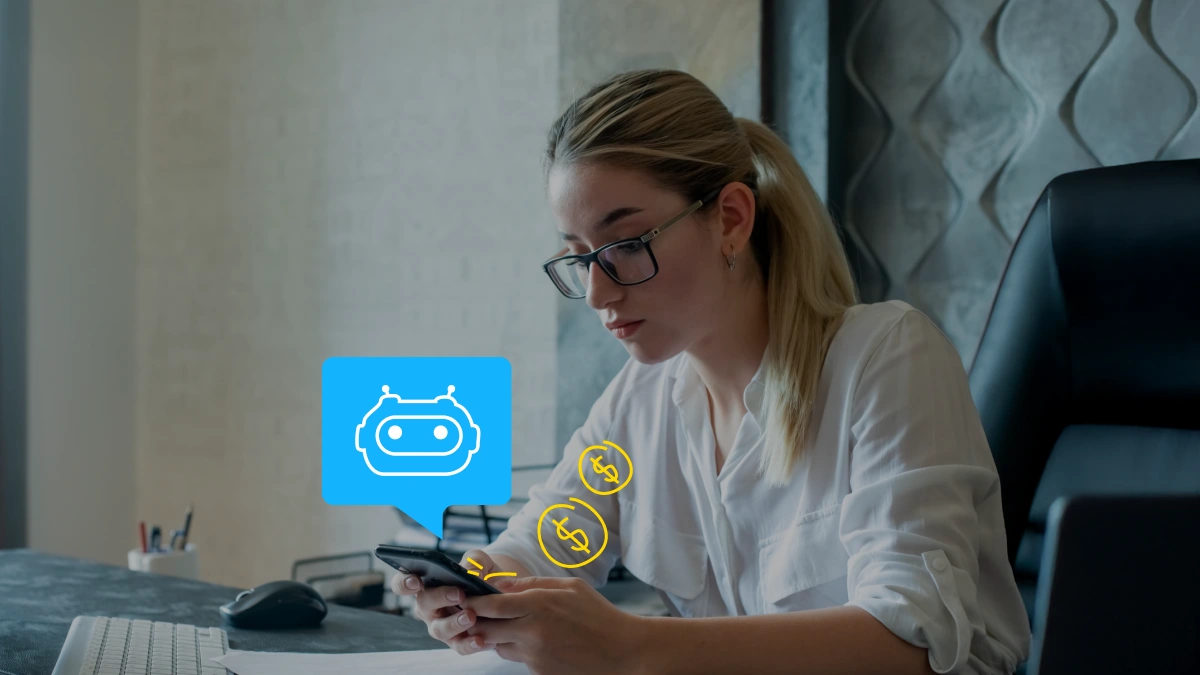
The adoption of Artificial Intelligence in banking has become a game-changer, which is expected to drive the way financial institutions provide seamless and personalized experiences to their clients. Based on the recent research by Markets & Markets, the conversational AI banking sector will show nearly triple growth in the next 3-4 years, increasing from $10.7 bn (dated 2023) and reaching $29.8 in 2028.
New AI technologies offer a fundamental shift in banking products and services, providing users with dynamic personalized interactions. Using ML (machine learning), advanced conversational analytics, and NLP (natural language processing), AI in the banking industry has reshaped the customer journey. It empowers financial organizations to deliver automated banking tasks and tailored customer experience across different touchpoints – like chatbots and conversational AI in banking and financial assistants giving real-time solutions, or providing guidance through comprehensive financial processes.
The Impact of Conversational AI for Banking
The implementation of conversational AI systems in online banking and financial services is a result of businesses looking to (1) improve customer experience, (2) automate operations and boost efficiency, and (3) remain competitive as the digital landscape is rapidly changing and offering more and more new opportunities, along with challenges.
Technology keeps developing, so financial organizations need new ways to further improve their personalized services. Today, many banks are using conversational banking to bring the impact through the following capabilities: Speech Recognition, Content Generation, Language Understanding, Summarization, Document identification, and Knowledge Assist.
Speech Recognition
The efficiency and accuracy of automated voice-based interactions have been significantly improved by AI-powered speech recognition. Just as it has been before, users can interact with their banking providers via voice commands, for example, to make payments, check account balances, or get personalized assistance.
But now the whole range of services like virtual advisors, IVR (interactive voice response systems), and voice-controlled conversational in banking interfaces have become even more personalized and convenient banking features .
Content Generation
Generative AI and language models for content generation can streamline the process of communication through different content formats (e.g. product descriptions, SMM posts, articles, and other marketing materials). However, it’s still important to recognize types of content and situations when a human agent is better suited.
For example, balancing AI-generated content / responses for simple routine requests / topics with human agents for more personalized and complex issues.
Eventually, Artificial Intelligence can help businesses engage customers more effectively, just remember about transparency – inform users when they are interacting with AI and offer an option to go for a human operator if needed.
Language Understanding
Natural language processing technology powered with conversational AI is revolutionizing the realm of automated language understanding (NLU), making it possible for systems to interpret human language with unprecedented accuracy.
This includes contextual understanding (models that recognize customer intent and preferences), continuous learning (the use of Machine Learning algorithms to adapt to changing customer needs and language patterns), and personalized interactions (the use of account history to provide tailored responses, which is especially effective for recommendations of products and services or personalized financial advice).
Summarization
AI-driven algorithms can be extremely helpful for users when it comes to generating easily digestible and concise summaries of lengthy financial documents or conversations. This is especially useful for customers who need to understand important information, such as terms of service, complex contracts, or loan documents.
On the other hand, such concise summaries of the client’s interaction and conversation history can help human agents or contact center representatives better understand the issue and provide an optimal solution.
Document Identification
Machine Learning algorithms used for AI-based document classification can help automatically analyze and classify different types of documents and interactions, such as forms, contracts, or emails. Here conversational AI ensures a streamlined process of document management and greatly improves efficiency by reducing manual effort and eventually cutting transaction time for end-users.
Knowledge Assistance
Knowledge management systems powered with NLP technologies and ML algorithms can effectively retrieve, organize, and categorize data from support documentation, internal knowledge bases, regulatory guidelines, and FAQs. Conversational AI can also help frontline customer support, as well as automated self-service tools get instant access to up-to-date and accurate information.
Key Benefits of Conversational AI in Banking
The rise of conversational AI in financial services can offer numerous benefits for banking organizations (greater efficiency, data analytics, and competitive advantage through innovation), and make things easier for clients too.
Benefits For Banking Organizations
Cost savings & Efficiency | Conversational AI helps banks effectively optimize operational costs related to manual customer support and customer engagement. Banking chatbots can cope with a high volume of interactions at the same time, free up resources, and make it possible for staff to focus on more important and value-adding activities. |
Analytics & Data Insights | By processing huge volumes of data from customer interactions, conversational AI enables invaluable insights unavailable so far. Banking institutions can use customer preferences, common pain points, and trends to optimize product / service offerings and adjust growth and retention strategies accordingly. |
Competitive Advantage | Implementing AI solutions on time shows banks as innovators and leaders in the industry, contributing to a stronger brand name. Eventually, they can bring in new customers, keep existing ones, and get the differentiation necessary to survive in a highly competitive market. |
Benefits For Users: 5 Customer-Centric Use Cases of Conversational AI
Making Payments | Conversational AI chatbot can help clients through the purchase process and provide assistance with selecting the right payment options. Such AI assistants are faster and much more convenient than using a website or banking application to make payments. |
Analyzing Charges | Virtual assistants can help users easily access information on recent transactions and charges. They provide greater control over active subscriptions and highlight the ones which are to be paid or are no longer required. |
Securing Funds Transfer | AI-powered chatbots offer a safe way of transferring funds between accounts. By monitoring transaction history, checking user location, and asking user-specific questions, these solutions contribute to reducing potential fraudulent activities. |
Getting Product Offers | Bank clients can easily get summarized information about loans, interest rates, and other latest information like exchange rates. Virtual assistants are connected to the banking organization’s databases and provide one-stop access to available banking services, helping users make more informed decisions. |
Taking Emergency Action | Modern solutions can help users immediately lock or freeze a credit card account (for example, whenever the card is lost / stolen, or sensitive data seems compromised to third parties). Now there is no need to call the banking provider directly and wait for support to manually process a request. |
Implementing Conversational AI in Banking and Finance Services:
Best Practices
To implement conversational AI solutions and get the best ROI, financial organizations need to follow a strategic approach that would raise the customer experience to a qualitatively new level. This will also drive higher operational efficiency by offering maximum personalized, automated, and fully available service to clients. Below are the best practices that will help you achieve measurable business impact.
Set Clear Strategic Objectives
First, you should define clear objectives – build them around use cases and customer pain points. Make your conversational AI initiatives aligned with strategic priorities like improving customer service, boosting sales and marketing, and growing operational efficiency.
For example, you can integrate real-time fraud detection alerts, use conversational AI to streamline document submissions and eligibility checks, or offer personalized financial guidance, including budgeting and spending analysis.
Select Vendor & Technology
Next, it’s crucial to select the right vendor and technology. Evaluate shortlisted candidates focusing on their tech capabilities, scalability, security standards, and regulatory compliance. The platform’s NLU (natural language understanding) should handle specific financial terminology, accurately detect intent, and support multiple languages.
Look for scalability and solutions that can be deployed across multiple channels like mobile banking apps, websites, chat, and IVR systems. Focus on vendors that offer flexible options, including cloud, on-premise, and hybrid models to fit your specific needs.
Don’t forget to assess security and compliance features. Check whether the vendor’s solution meets industry-standard encryption and data protection practices, including secure data storage and transmission. Ensure that conversational AI solution complies with financial regulations like GDPR, PCI DSS, and CCPA.
Mobilunity can be a strong choice for fintech software development due to our deep financial sector and banking experience, compliance knowledge, global talent sourcing capabilities, and flexibility in providing specific solutions tailored for complex, regulated industries.
We have a track record of successful projects in the fintech space and AI use cases in banking. For instance, we supported a Canadian financial company with a dedicated DevOps team, assisted a Spanish bank with UX/UI design improvements, and helped a payment consolidation system which needed to streamline user payments from Amazon and other platforms for cost savings. Each of these projects involved adapting to complex financial requirements, integration of industry-specific tech stacks, and strict compliance standards.
Establish Seamless Integration
Seamless integration with core banking systems, CRM systems, and legacy systems is important for the smooth and successful adoption of conversational AI applications. You should select a solution that supports seamless data transfer, transaction processing, and real-time access to information.
Look for comprehensive API and SDK availability – this will help you with flexible customization, and make it easier for your development team to integrate and adjust the system as necessary. Also, if you’re using legacy systems, ensure the conversational AI offers smooth integration (or that the vendor has strategies for phased or gradual integration).
Ensure Customization & Train Models
To maximize operational efficiency, it’s critical to fine-tune and train conversational AI models, based on customer preferences and specific use scenarios. The solution should allow access to customer data, such as transaction history, to personalize recommendations, financial guidance, and support responses.
Focus on platforms with highly customizable workflows that allow adjusting conversation flows and integrating conversational AI technologies with your internal systems like CRM or banking core. If your organization operates across different regions, the solution should support multilingual capabilities and region-specific customizations.
Arrange Performance Monitoring
Rich analytics and reporting capabilities are essential for allowing continuous improvement and correcting issues quickly. Your conversational AI platform should offer real-time analytics on key metrics like user engagement, response accuracy, and query resolution rates.
Look for a solution that provides sentiment analysis and customer feedback capture, enabling you to identify pain points and optimize the system. You should leverage analytics and reporting dashboards for ongoing monitoring of usage patterns and interactions, as well as KPI tracking (e.g. transaction completion rates, query resolution rates, response accuracy, etc.). Doing so, your team will be able to quickly identify pain points and optimize performance.
Bottom Line
A strategic approach to the implementation of conversational AI in the banking has the power to change the financial services industry. A well-supported AI-based solution seamlessly integrated into a banking application can significantly increase business efficiency. Banking institutions need greater cost savings and higher operational performance, powerful analytics with invaluable data insights, and a competitive advantage unavailable so far – that’s where conversational AI comes into play. For end-users, the power of AI implemented through chatbots and voice assistants can streamline payments, secure and speed up transactions, and provide a generally more intuitive and enjoyable experience.
The future of conversational AI is dynamic and exciting. While conversational AI offers numerous benefits – both for users and financial service providers – most organizations aren’t too active to take on full investment in AI-driven solutions. However, early adopters of this technology will have a critical advantage in such a competitive market. So if you’re looking how to initiate or speed up the integration of conversational AI in your project, you may need additional development resources. Check out Mobilunity’s professional fintech developers for hire and related case studies in the digital banking industry.